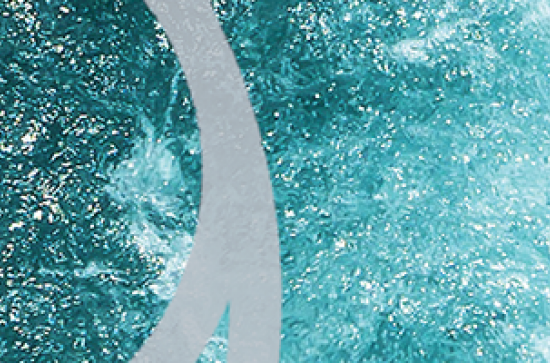
https://us02web.zoom.us/j/88018185733?pwd=OEcxb2ZSYUwzV2hWTE9wcE1yTWRUQT09
Passcode: 279596
Speaker: Professor Josephine Sullivan, KTH Royal Institute of Technology, Stockholm
Title: "Are All the Linear Regions of a ReLU Network Created Equal?"
Abstract: This presentation will describe recent research described in the paper "Are All Linear Regions Created Equal?" published at AISTATS 2022. The function represented by most ReLU neural networks is piecewise affine. The number of linear regions defined by this function has been used as a proxy for the network's complexity. However, much empirical work suggests, especially in the overparametrized setting, the number of linear regions does not capture the effective non-linearity of the network's learnt function. We propose an efficient algorithm for discovering linear regions and use it to investigate the effectiveness of density in capturing the nonlinearity of trained VGGs and ResNets on CIFAR-10 and CIFAR-100. We contrast the results with a more principled nonlinearity measure based on function variation, highlighting the shortcomings of linear regions density. Furthermore, interestingly, our measure of nonlinearity clearly correlates with model-wise deep double descent, connecting reduced test error with reduced nonlinearity, and increased local similarity of linear regions.
Bio: Josephine Sullivan is an associate professor at KTH Royal Institute of Technology, Stockholm within the school of Electrical Engineering and Computer Science. She has worked for several decades within the field of computer vision and seen the field transformed by deep learning. Her initial research focus was on object and multi-target tracking in visual data and 3D human pose estimation. More recently she has performed research on representation and transfer learning and multi-modal learning between vision and text. She received her PhD from Oxford University (eons ago) and before that a BA in Mathematics from Trinity College Dublin (even more eons ago).